Since the release of ChatGPT by OpenAI in November 2022, followed by several other massive artificial intelligence (AI) bots, there’s been a renewed focus on how AI is permeating every aspect of our lives. In the leadership industry, one of the hot questions we’ve been hearing is whether we can trust AI-based leadership assessments.
These questions about using AI in assessment aren’t new. While the release of new AI bots has sparked attention, industrial-organizational psychologists, HR leaders, and legal departments have been grappling with questions about AI for more than a decade.
There isn’t consensus about the use of AI in workplace assessments. But most assessment developers understand the power and capabilities that AI could provide, while understanding the need for due diligence to ensure that the risks don’t outweigh the benefits.
AI-based leadership assessments could provide novel enhancements to traditional assessment (e.g., cost savings, enhanced prediction) if deployed correctly. But the impact could be disastrous if you don’t address the risk factors of AI.
As AI becomes more established in the workplace, it is crucial to balance the potential benefits with the need for transparency, accountability, and ethical considerations. In this piece, I’ll cover the top concerns that HR and company leaders should consider as they look at using AI in leadership assessments.
When and Why to Use Assessments for Leaders
Before discussing AI further, let’s level set on the goals and uses of assessment. At DDI, our mission is to hire, promote, and develop exceptional leaders who lead others toward a better future, and we recommend and use assessment to aid in these efforts.
Pre-hire and post-hire assessments can be valuable tools for organizations to improve their selection, promotion, and training procedures. Pre-hire assessments can be used to evaluate a candidate's skills, capabilities, knowledge, personality, and work styles. They help to ensure that a candidate is a good match for the position and the organization's culture while also using key job criteria to predict performance in the role. These assessments can also help to identify areas for concern, such as lack of effective behavioral intervention in a simulation to aid the decision-making process of selecting the ideal candidate.
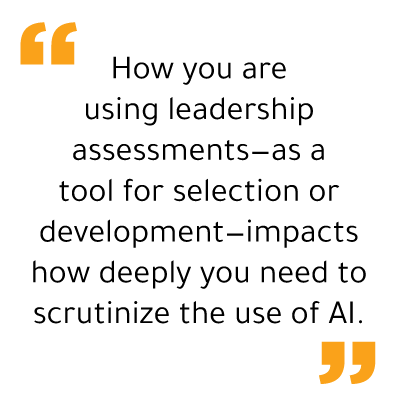
Post-hire assessments are used to evaluate employee performance and provide feedback for development and training. These assessments can be used to identify areas where an employee is strong in performance (the “glows”) and where they may need additional support (the “grows”), such as training or coaching. Post-hire assessments can also help to identify potential issues before they become major problems, such as low productivity or job dissatisfaction.
How you are using leadership assessments – as a tool for selection or development – impacts how deeply you need to scrutinize the use of AI. While you need to be judicious in how you use assessment for development, assessments used to make employment decisions have to stand up to a higher level of scrutiny. They need to feel fair to the candidate and reassure the hiring manager that they accurately predict performance. And critically, they need to also stand up to potential legal scrutiny.
The Potential of AI in Leadership Assessment
Given the care with which we must handle AI, some people wonder if we should be using it at all in assessments. However, the potential benefits are significant. The use of AI in leadership assessments has the potential to revolutionize the way we identify and develop effective leaders, creating better outcomes for organizations and society as a whole.
Without hyperbole, the opportunities are beginning to look endless for the advantages of using AI to complement your assessment programs. From a practical perspective, here are some of the potential benefits of AI-based leadership assessments:
- Increased cost savings for developing, scoring, and administering assessments.
- Increased efficiencies to enhance the candidate experience (e.g., reduced administrative time of an assessment solution) and administrator experience (e.g., immediate results from your candidates).
- Enhanced capability to incorporate numerous data points to increase consistency, reliability, and validity of the results for nearly maximum utility of an assessment solution.
- Ability to reduce biases and subjective ratings to decrease adverse impact risk and measurement error.
The powerful advantages offer a strong incentive to apply AI technology for better results. The challenge, of course, is how to optimize these benefits while reducing the risks.
5 Top Concerns of Using AI in Hiring and Assessments
The increasing use of AI-based leadership assessments has sparked concern in many ways. Many of these concerns have reoccurred over time with each new advance in technology. The good news is that the industry has rapidly evolved to create new standards that combat these concerns. However, it’s important to continue asking these questions and paying attention to how assessment providers respond.
Here are several of the most common concerns:
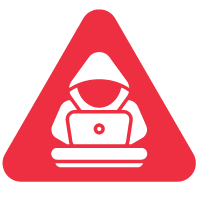
1. Data Privacy and Security
One of the primary concerns with using AI technology is protecting individuals’ private data. Often, people are unaware of what data is being collected about them and how it’s being used, which is a major problem if that data could affect their employment.
For example, a prominent AI-based recruitment and selection tool company recently faced legal action from the Federal Trade Commission (FTC) in the U.S. for allegedly violating participants' privacy rights. According to the lawsuit, the company used AI to analyze candidates' facial expressions, tone of voice, and other non-verbal cues during video job interviews.
Candidates say that the company did not obtain proper consent or inform them of the extent of the analysis. Since the lawsuit was introduced, the company sunset these features from their product.
This example shows the potential of AI in predicting workplace performance, but also the risks of deploying new technologies without appropriate regard for legal implications.
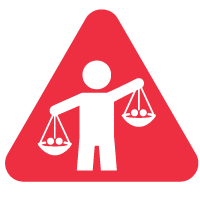
2. Bias
Bias is one of the most common and concerning issues facing AI programs. Algorithms learn using existing systems and data. If bias is baked into existing systems, the resulting algorithm will likely also show bias. As the saying goes, “bad data in, bad data out.”
There are a number of ways to train algorithms to be less biased than existing systems. For example, it's essential to have a diverse and representative training dataset. In other words, the data should accurately reflect the makeup of the real-world population that the algorithm is intended to measure. The training data should include a range of different genders, ethnicities, ages, cultures, and socioeconomic backgrounds. In addition, preprocessing or cleaning the training data is an essential step to account for any missing values, errors, significant outliers, or irrelevant data that could bias the algorithm’s output. However, with every advancement in AI, there are new opportunities to introduce bias to the system. That’s why it’s important to tie AI-based measures to job-based criteria and regularly test, monitor, and evaluate your algorithms for bias in high stakes applications, with frequency beyond typical or classical assessment monitoring.
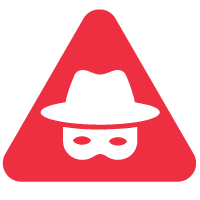
3. Cheating or Faking
Cheating or faking answers has always been a concern, even before assessments played a role in decision-making processes with more focus on cheating/faking with the transition from pen-and-paper on-site events to computers. In the mid-2000s, practitioners and researchers responded with research that drove standards and recommendations, spurring the best practices for today’s online assessment standards, known by the unwieldy term “unproctored internet testing” (UIT). Strategies like time limits, virtual proctoring programs, etc. helped to safeguard the integrity of candidate responses.
Fears of cheating and faking are renewed now that AI can provide responses to open-ended items. However, there are already methods in progress to combat this challenge. For example, forced-choice personality elements can combat an AI approach to fake a test. As AI advances, so do industry approaches to prevent cheating.
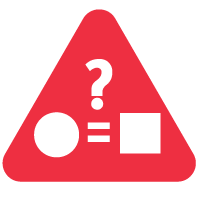
4. The “Black Box” Problem
“Black box” describes the ability of AI to show that two things are correlated without being able to explain why. I would argue that this is one of the most novel and concerning challenges with AI.
A famous example of black box occurred when researchers were trying to train AI to identify skin cancer. They fed the system many images of malignant and benign skin lesions and found that the algorithm was able to correctly identify which were malignant.
But how did it know? Upon a closer look, the researchers discovered that the algorithm determined that any picture of a lesion that also contained a ruler indicated malignancy. That’s because rulers are always included in medical photos of malignant lesions to show the size of the lesion. But to the algorithm, the mere presence of a ruler in the image likely indicated cancer.
This flawed logic is one of the most potentially damaging challenges of AI. With leadership assessments, it’s crucial to be able to explain how models predict the competencies or scores in our assessment and that can be observed in our technical documentation. Any HR practitioner should be extremely wary of using any assessments for which the “how” and “why” of the results cannot be explained.
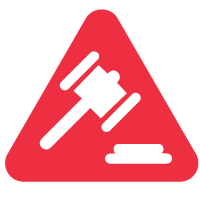
5. Legal Defensibility
All of these challenges culminate in a larger concern: legal defensibility. Local, state, and federal governments are rapidly enacting new laws to protect individuals’ privacy and equal opportunity to work.
But the rise in lawsuits doesn’t mean that companies shouldn’t use assessments to evaluate candidates. In fact, using data correctly can help to reduce bias in the process. It means that you have to understand exactly what you are assessing, how the assessment works, and how it’s related to the jobs you are hiring for. I can’t emphasize it enough: you need to be able to provide thorough documentation of the development and scoring of an AI-powered assessment.
Criteria for Using Leadership Assessments with AI
Like every other innovation that comes to market, we should move forward with cautious optimism. Technology is only as powerful as how we choose to apply it. In this section, I’ll cover some of the key criteria you should use when considering an AI-based leadership assessment.
For those who want a more technical deep-dive into using AI-based assessments, I highly recommend reading the recent guidelines issued by the Society for Industrial-Organizational Psychology (SIOP). At DDI, we follow these guidelines to develop and employ AI-based leadership assessments.
If you don’t want to wade through the technical recommendations, here are a few high-level questions we recommend asking when you consider assessments that may include an AI component:
- What types of objective data are you collecting? You can collect many types of data, some of which are more reliable than others to predict job performance. Here’s a quick overview of the types of data known as “signs versus samples.”
- How are you measuring validity and reliability? An assessment must be both valid (i.e., it accurately measures what it was designed to measure) and reliable (i.e., it provides similar scores for people with the same level of a characteristic). Any assessment provider should be able to answer these questions and provide documentation.
- Do you use human assessors, and if so, how do you train them? Some assessment providers also use human assessors – as DDI does – in conjunction with AI. This combination of human and technology-based assessment is a powerful way to mitigate bias and deliver a more nuanced and holistic view of a person’s capabilities. However, you need to ensure that assessors undergo appropriate training.
- How do you apply quality control? Assessments need to be offered in a consistent way, with minimal differences across different delivery methods, such as browsers, hardware, etc. It’s also critically important that they meet accessibility standards.
- How are you minimizing bias from machine learning? The assessment developer should be able to explain what steps they are taking to combat bias in their algorithm.
- How are assessments monitored and maintained? Over time, assessment developers need to regularly monitor their data for ongoing signs of bias among groups, including those with disabilities. In addition, you need to ensure that you update global and regional norms as necessary to make fair comparisons.
While this list isn’t comprehensive, it’s a good start to think about what questions to ask your assessment developer.
Data Is Only as Good as How You Use It
Data does nothing when it sits on the shelf. It’s all about how you use it.
If you are using data to make any leadership decisions, I’ll leave you with a few guiding principles:
- It’s all about job relevancy. This must be your guiding principle above all else. Every piece of data you consider must be directly relevant to the job the person is being asked to perform – or you shouldn’t use it. Importantly, especially when it comes to AI, you need to be able to explain why and how the data relates to performance. You can’t accept the “black box.”
- Assessment should be one source of information among many. Assessment data should inform decision making, not dictate outcomes. The results of an assessment must be reviewed, discussed, and integrated with other information, such as job performance, behavior on the job, personal attributes, etc. It should not be the sole information used to make a decision.
- Data is part of a structured dialogue. One of the biggest mistakes we see companies make is talking about and using data inconsistently, which can lead to bias. Ensure that you have a structured process for talent review discussions that incorporates data consistently.
- Remember that behavior rules. Assessments can measure many aspects of a person. Some of these things, like personality traits, can give you insight into what a person is likely to do or what motivates them. But ultimately, leaders’ behavior and choices will determine their performance on the job. So take care to think holistically about how you value personality assessments compared to behavior.
Keeping Pace
AI technology is advancing at an unprecedented pace, and we feel that impact everywhere. But technology is developing faster than we can critically evaluate it, creating a significant challenge for businesses and individuals who are trying to keep up with the latest advancements in AI.
It is important to adapt to new technology and embrace its potential, but it is equally important to think carefully about the impact of AI, especially when it comes to decisions that affect people's lives. In March 2023, there was a call to action from business leaders, researchers, and philosophers seeking a six-month pause on the development of AI beyond the GPT-4 model to allow the world to catch up to the development of AI.
While others debate the use and regulation of AI, it’s up to us to leverage the opportunity while minimizing or eliminating the risks. When used well, we believe that using AI in assessments can help companies select and develop better leaders with less bias. To do that, AI leadership assessment systems should be transparent, explainable, and unbiased.
Moreover, businesses should be careful not to rely entirely on AI technology and overlook the human factor. Having checks and balances between AI models and human decisions can lead to much better outcomes than using technology or human discretion alone. The decisions made by AI should always be checked and verified by humans to ensure their validity and fairness.
Ultimately, AI technology is and will increasingly be a valuable tool for assessments, but we should approach implementation responsibly to ensure that it serves the best interests of all stakeholders involved.
Learn more about Leadership Assessments with DDI’s Ultimate Guide to Leadership Assessment.
Chris Coughlin is the Manager of Assessment Content Development and Design at DDI where he leads a team of scientists that develop, test, validate, QA, deploy, and maintain innovative assessments for leadership selection, leadership learning and development, and executive solutions. He enjoys coaching his daughters at soccer and math, working as the guinea pig in the batter box for his son’s fastball, and attending team trivia matches at local restaurants with his family. Finally, Chris enjoys playing Catan with family and friends (and steadfastly proclaims that Catan is the best board game of all time) during relaxing weekends.
Topics covered in this blog